Descriptive analysis and in-depth exploration of quality and production data are used to understand industrial processes and machine control rules in order to optimize production and anticipate defects.
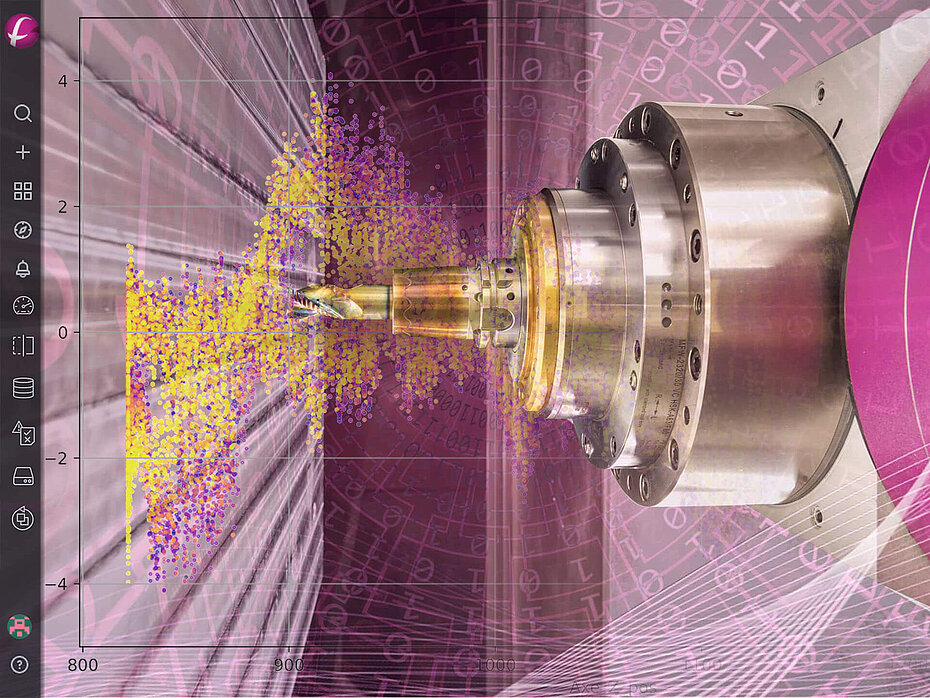
QUALITY MEASUREMENT - SPC
Railway sector
Objectives:
- Control production quality by automated processing of machine data.
- Improve the management of data generated during the manufacturing process.
The Solution:
- A tool for creating control charts to define thresholds and alert channels.
- Manual qualification of defects by a competent operator or manager and instant analysis of non-quality causes.
Benefits :
- Quick consultation of the status of alerts and receipt of important notifications by email.
- Quality indicators updated in real time.
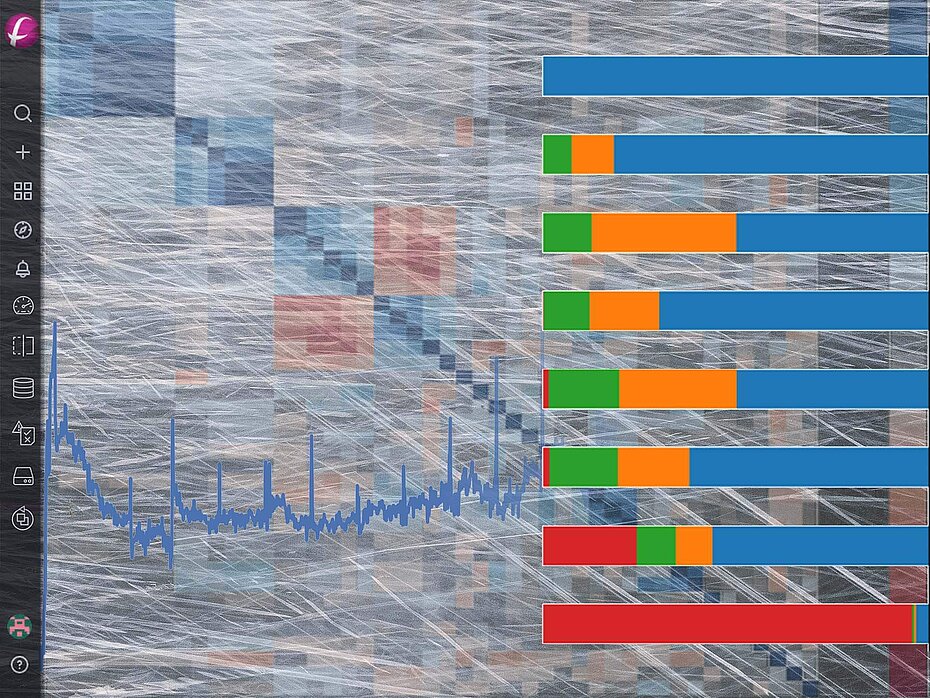
ANALYSIS OF THE CAUSES OF NON-QUALITY
Self-adhesive protective film production sector.
Objectives:
- Objectivize the causes of non-quality and produce recommendations to significantly reduce rejects (>10% at the start of the study).
The Solution:
- Analyze the process with the business and consolidate a large quantity of data from production machines and quality control.
- Produce an exploratory analysis to study the correlations and dependencies between the data.
- Identify all factors influencing quality and model their impact.
- Synthesize the findings, consolidate the conclusions with the client and formulate recommendations through a quality improvement plan.
- Evaluate the possibility of deploying an online quality prediction model.
Benefits:
- By implementing our recommendations and reviewing the quality control accordingly, the reject rate was reduced by 50%. A result that led our client to call on us to further optimize its processes.
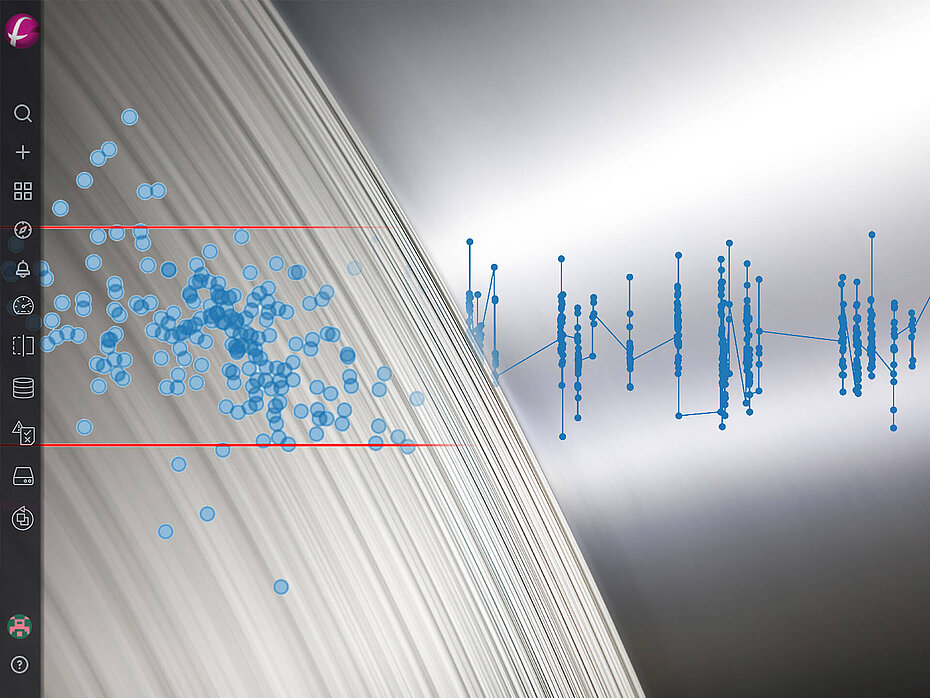
PREDICTIVE ANALYSIS OF STEEL PROPERTIES
Carbon steel production sector - Galvanizing line.
Objectives:
- Control and adjust in real time the critical parameters to avoid any quality degradation at the end of production.
- Optimize production costs and maximize performance by avoiding end of line destructive tests and the downgrading of finished products.
The Solution:
- A hybrid physical / Machine Learning model and a process parameter optimization loop that provides alerts on drifts and adjusts the operation if necessary:
- Predict the mechanical properties of steel taking into account multiple influencing factors (>1000).
- Ensure the repeatability of results for a new product, with poorly controlled physico-chemical properties, in a context of significant variability of raw material quality.
Benefits :
- Improved accuracy in the evaluation of the main characteristics of the steel produced (*2 to *6 !!!) during production.